Trusting AI to Reveal The Precious Jewels of Document Review
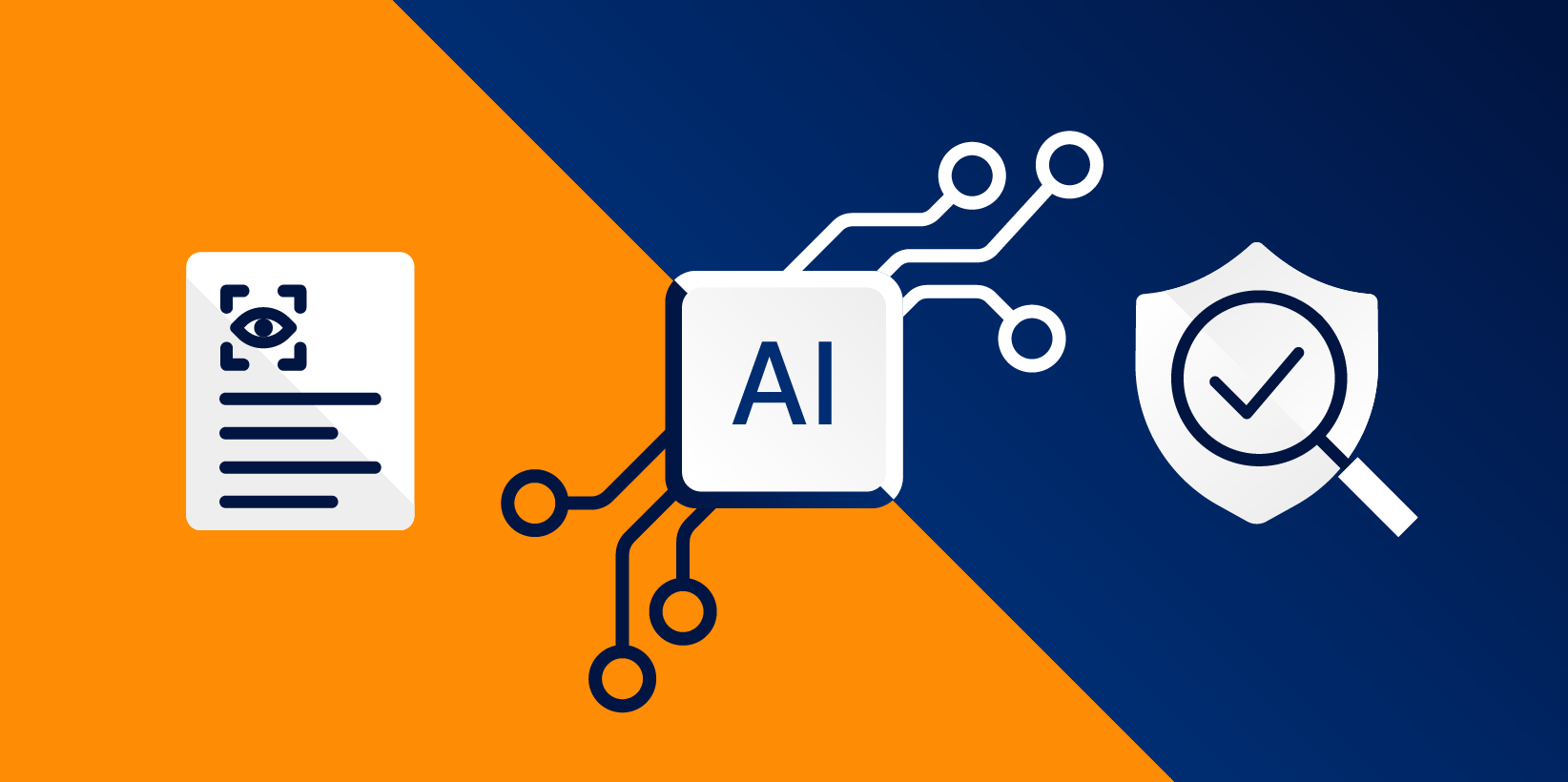
-min.jpg)
The document review phase of eDiscovery is critical for unveiling insights, shaping strategies, and influencing the trajectory of legal cases. It reveals responsive documents that uncover pivotal evidence, recognize vulnerabilities, and reveal patterns that determine case outcomes. And increasing data volumes and complexity mean that review teams have more than ever to cover.
With stakes this high, missteps during document review can lead to missed insights, damaging disclosures, or costly legal consequences. Legal teams know they cannot compromise precision, security, and quality. But, at the same time, they need more efficient, cost-effective ways to wade through troves of data to identify relevant information.
Many legal teams see AI's potential and recognize its advantages. However, they struggle to fully trust it, which leads to the creation of the “AI Trust Gap.” This gap stems from concerns about AI’s reliability, especially compared to traditional, human-driven review processes. AI tools, particularly generative AI, are known to produce errors like hallucinations—where they output misleading or incorrect information—and inconsistencies from even subtle changes in input. These risks have left legal teams lacking confidence and wary of relying on AI, especially in high-stakes matters where precision is essential.
As we consider generative AI-assisted review (GAR) as an option, ensuring AI document review experts remain in control allows teams to attain the accurate outcomes that they need. In this process, experts lead AI tools through six steps to deliver consistent results that review teams can trust.
Step 1: Assessing the dataset
Generative AI excels at analyzing large volumes of data quickly. When applied to document review, it can rapidly assess datasets to detect relevant legal issues, ambiguous language, or sensitive content, then suggest whether and why a document may be responsive. However, by itself, generative AI may fail to fully understand context, leading to misclassification and gaps in analysis.
This is where human expertise becomes invaluable. Experienced professionals can guide GAR’s initial assessment, defining and refining the prompts and assessment setup to ensure the AI model focuses on the right objectives and issues. Humans add insight that AI cannot achieve independently, identifying subtle risks and ensuring the assessment is accurate from the start, thus laying a more reliable foundation for the review.
Step 2: Designing the review
Generative AI’s capabilities in identifying patterns in large datasets give it the potential for streamlining the review. However, maximizing this potential necessitates thoughtful integration into the broader project design. And to do that, human expertise is needed.
The primary goal is to ensure that the AI enhances the review, driving efficiency without sacrificing quality or accuracy. To achieve this goal, experts must carefully establish project objectives, define the review’s scope, and select AI tools that, with clear documentation to explain outputs, are transparent and able to demonstrate how they arrive at decisions. Without this clarity, AI can lead to inconsistent results and widen the trust gap.
It takes expertise to make this design process work effectively. Experienced review managers and analytics experts, who possess extensive document review experience and legal domain expertise, guide the AI model by determining the appropriate protocols for the review, ensuring that it is trained and refined to focus on the right objectives. These experts are needed to oversee the AI and ensure it aligns with the complexity and nature of the documents in question.
Expert-led GAR isn’t just about experts controlling and manipulating AI. It’s about integrating AI into the process thoughtfully to deliver the best, most efficient review experience. In this hybrid approach, AI assists with the heavy lifting while human experts control decision-making, ensuring every review is conducted with the required precision and oversight. This collaboration builds confidence in the process, allowing legal professionals to trust the outcomes generated by the AI.
Step 3: Executing the review
Generative AI can process vast amounts of data with impressive speed. It automates the initial review work, assigning relevance scores to documents. However, AI models need more depth of understanding for complex, multifaceted cases, as they rely on predefined patterns and algorithms that may not understand deeper meaning or context.
For instance, if emails include discussions about a potential business partnership, GAR might struggle to understand subtle distinctions in intent between casual negotiation language versus legally binding commitments.The AI might misclassify phrases like "let’s move forward" as a definitive agreement. However, a legal expert would understand that the parties may still be in the negotiation phase.
AI can also fail to capture cultural or organizational jargon, sarcasm, or indirect language that a human reviewer would immediately recognize. Without the depth of understanding that comes from years of legal and document review experience, AI may overlook key evidence or misinterpret critical information, leading to errors that could impact a case's outcome.
We’ve coined the term “iterative prompting” to describe the ongoing engagement a document review expert, trained in AI, uses to drive this process. As the review progresses, they use their contextual understanding to guide the AI model to focus on increasingly nuanced legal questions, ensuring that multi-issue queries are addressed accurately. Through iterative prompting, human reviewers can steer the AI model to deliver richer, more relevant results with evidence to support its classifications.
This interaction between AI and human expertise leads to fast, thorough reviews, delivering reliable, consistent results that review teams can trust.
Therefore, human expertise is essential for the execution phase, both with the initial design and the continual refinement of the AI’s prompts.
Step 4: Validating the results
A unique feature of AI solutions, compared with TAR, is thatAI can deliver detailed explanations of which error protocol has caused a document to be flagged as responsive. Involving experts is important when reviewing these results and ensuring the validation processes are robust, accurate, and legally sound. Experts must interpret the AI’s outputs, cross-check them with control sets, and confirm that the final results meet legal requirements. Experts should apply established validation techniques like elusion testing and sampling to cross-check the AI’s results.
Regular quality checks and audits, and consistent communication of AI's strengths and limitations, help maintain confidence in GAR. The key is to convey that AI augments human expertise, and validation ensures that AI-generated results meet review teams’ expectations for accuracy and reliability.
Build trust in AI review with an expert-led solution
Generative AI is no longer a nice-to-have feature—it’s a necessary tool for managing the scale and complexity of today’s document reviews. However, without human oversight and insight to expertly lead it and guide it to the best outcomes, AI cannot deliver reliable results.
Guided AI Review pairs cutting-edge AI technology with human oversight, closing the AI Trust Gap and delivering results that legal teams can confidently rely on. Our AI Guides leverage their decades of experience to mitigate AI’s flaws, reduce the risk of inaccuracies, and optimize review workflows.
Read more about how Guided AI Review can enhance your next document review.