Validating GenAI-Assisted Review Results: Is That Your Final Answer?
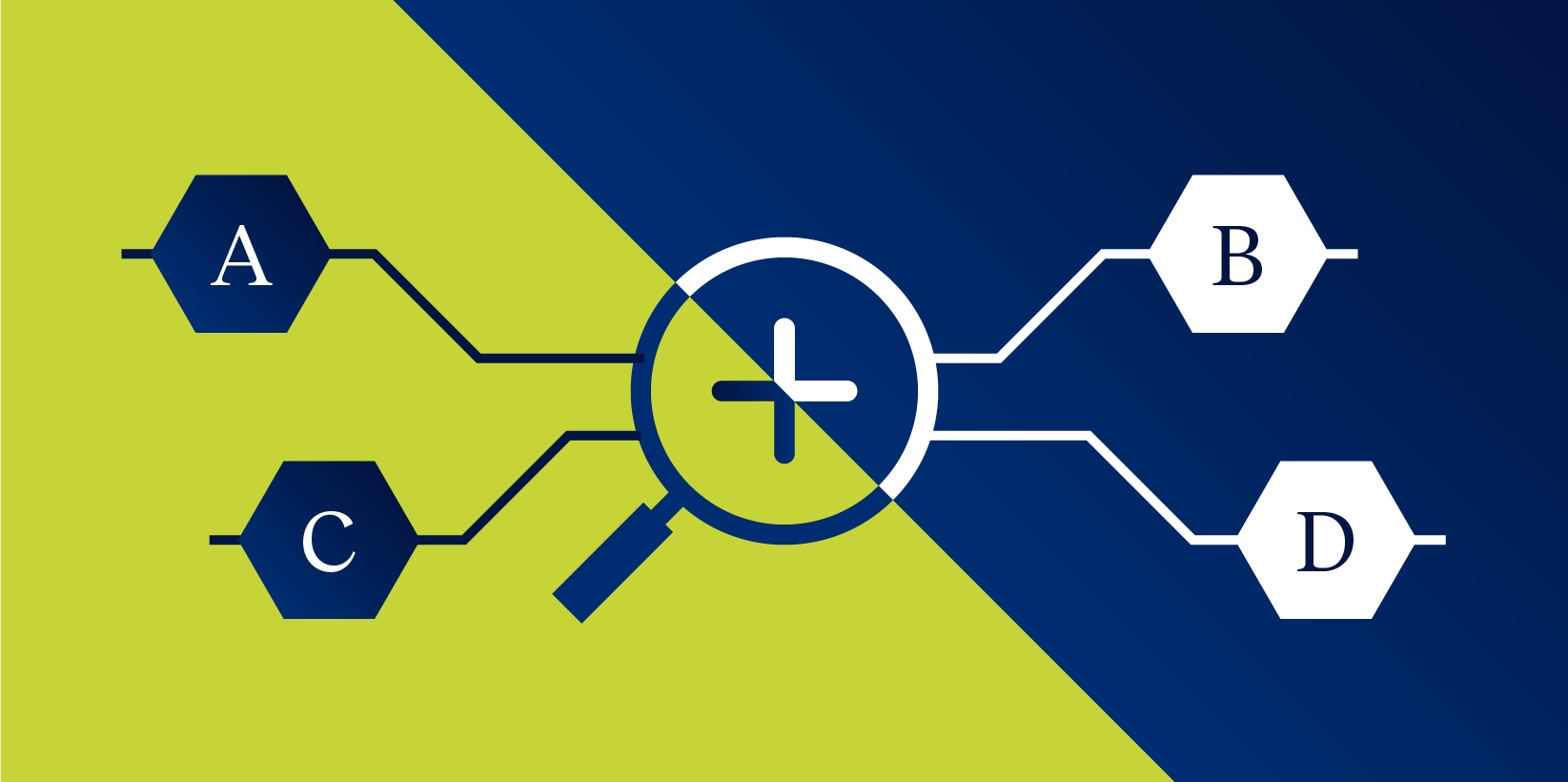
-min.jpg)
Whenever new eDiscovery technology appears, promising answers, the million-dollar question is always: how confident can you be about those answers? Attorneys must certify that any discovery response produced is consistent with the rules, and it has long been common practice to reach for one or more validation “lifelines” to check that the results of legal document review processes – however accomplished – are reasonably accurate and complete.
Generative AI (GenAI) tools take us beyond abstract, metaphorical “answers” to our questions represented by numerical scores and into a new era of literal answers in natural language. This apparent simplicity can be deceptive, however, and that seeming ease of use does not relieve us of our obligation to validate the results of our tools and ensure the quality of our processes. We must always take steps to validate its answers before we commit to our “final answer.”
Thankfully, in the context of GenAI-assisted review (GAR), the classifications of a GenAI tool can be validated by reaching for the same well-established lifelines currently employed for TAR workflows: sampling, control sets, and elusion testing when applicable.

Sampling Generally
In review workflows, sampling is used to ensure a reasonable level of quality and completeness is achieved. For our purposes, sampling comes in two flavors: judgmental sampling and formal sampling.
Judgmental sampling is the informal process of looking at some randomly selected materials to get an anecdotal sense of what they contain. For example, reviewing a random 5% or 10% sample of a particular reviewer’s work would be a kind of judgmental sampling, as would reviewing all the documents where a software classifier and human reviewers disagree. You’re not taking a defined measurement with a particular strength; you’re getting an impression and making an intuitive assessment of quality and consistency.
Formal sampling is just the opposite: you are reviewing a specified number of randomly-selected documents with the goal of taking a defined measurement with a particular strength. Typically, that measurement is either how much of a particular thing there is within a collection (i.e., estimating prevalence) or of how effective a particular search or reviewer or assisted-review tool is (i.e., testing classifiers). Classifiers are tested by measuring their recall (i.e., how much relevant stuff they found) and their precision (i.e., how much irrelevant stuff they included).
Control Sets
In order to accurately test a software classifier’s recall and precision, you must already know the correct classifications of the documents against which you are testing it. For example, to determine what percentage of the relevant material your software has found, you must know how much relevant material there was for it to find. Since it is not possible to know this about the full document collection without reviewing it all (which would defeat the purpose of using more efficient software classifiers), your software classifiers must be tested against a smaller control set drawn from the full document collection.
Control sets are typically a few thousand documents in size, and they are created by taking a simple random sample from the full collection (after any initial, culling by date, file type, etc.). The control set is then manually reviewed and classified by human reviewers so that the software classifier can be tested against it. It is important that the review performed on the control set be done carefully and by knowledgeable team members, as small errors in the control set can be multiplied into large ones in the full review.
Elusion Testing
When using a software tool as a classifier to eliminate irrelevant materials from the review population, sampling can also be used to test the unreviewed population for any important materials that may have been missed. This is called elusion testing (measuring the quantity of relevant materials that eluded your classifier). Mathematically, elusion testing is just performing a standard prevalence estimation specifically on the unreviewed set of documents (sometimes referred to as the null set) to estimate the number of relevant documents in that set, if any.
There is no way to perfectly identify and produce all relevant electronic materials – and, thankfully, no legal requirement that you achieve such perfection, but there can be great value in being able to say with some certainty how little (or how much) has been missed. A reliable estimate can provide concrete evidence of the adequacy or inadequacy of a completed classification process, whether it was completed by humans, by searches, by TAR tools, by GenAI tools, or by some combination thereof.
Key Takeaways
GenAI tools’ ability to provide actual answers in natural language, but that seeming ease of use does not relieve us of our obligation to validate the results of our tools and ensure the quality of our processes – to be sure before committing to our final answer. Thankfully, in the context of GAR, the classifications of a GenAI tool can be validated by reaching for the same well-established lifelines currently employed for TAR workflows: sampling, control sets, and elusion testing when applicable.